Utilizing machine learning to create a blood-based scoring system for sepsis detection
Abstract
Introduction and aim. Sepsis, a disease caused by inflammation as a response to infection, often goes undiagnosed due to its heterogeneity and lack of a single diagnostic test. Current sepsis detection scoring systems have low sensitivity and utilize biomarkers that are difficult to obtain from a single test. The goal of this research is to create a scoring system that outperforms current industry standards by utilizing blood-based biomarkers readily available in hospital settings.
Material and methods. Machine learning algorithms were run through Google Colab using Extreme Gradient Boost classifier. The dataset was obtained from NCBI website containing electronic hospital records of intensive care patients. A multivariate linear regression was applied to the dataset to determine statistically significant biomarkers in the detection of sepsis, and their β coefficients. Then, validation testing was performed, and the performance was compared to other scoring systems.
Results. This experiment reveals that a sepsis detection system that utilizes procalcitonin, white blood cells, C-reactive protein, neutrophil to lymphocyte ratio, and albumin can outperform other biomarkers and scoring systems with high sensitivity at a recall score of 0.7922.
Conclusion. These results demonstrate the potential of utilizing a blood-based scoring system for sepsis detection within hospital settings.
Cite
Aref S. Utilizing machine learning to create a blood-based scoring system for sepsis detection. Eur J Clin Exp Med. 2023;21(4):830– 834. doi: 10.15584/ejcem.2023.4.27.
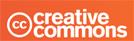
This is an open access article distributed under the Creative Commons Attribution License which permits unrestricted use, distribution, and reproduction in any medium, provided the original work is properly cited